
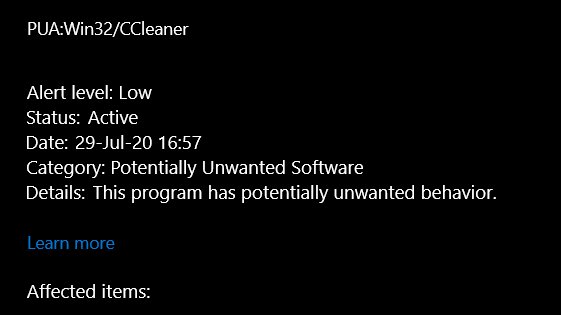
Our evaluation shows that the combination of different threat detection techniques within our framework improved detection capabilities.

We implemented our framework in an openly available prototype and demonstrate its applicability on multiple scenarios of the DARPA Transparent Computing dataset. This approach provides query options that facilitate contextualization over internal and external background knowledge, as well as the integration of multiple detection techniques, including tag propagation, attack signatures, and graph queries. KRYSTAL tackles these challenges by providing a knowledge graph-based, modular framework for threat detection, attack graph and scenario reconstruction, and analysis based on RDF as a standard model for knowledge representation.
Ccleaner malware threat report code#
The inflexible custom data models of existing prototypes and the implementation of rules in code rather than declarative languages on the one hand make it difficult to combine, extend, and reuse techniques, and on the other hand hinder reuse of security knowledge – including detection rules and threat intelligence. A key limitation, however, is that approaches developed so far are monolithic in their architecture and heterogeneous in their internal models. The result shows that LogKernel can hunt all attack scenarios among them, and compared to the state-of-the-art methods, it can find unknown attacks.Īttack graph-based methods are a promising approach towards discovering attacks and various techniques have been proposed recently. We evaluate LogKernel on the malicious dataset, which includes seven simulated attack scenarios, and the DAPRA CADETS dataset, which includes four attack scenarios.
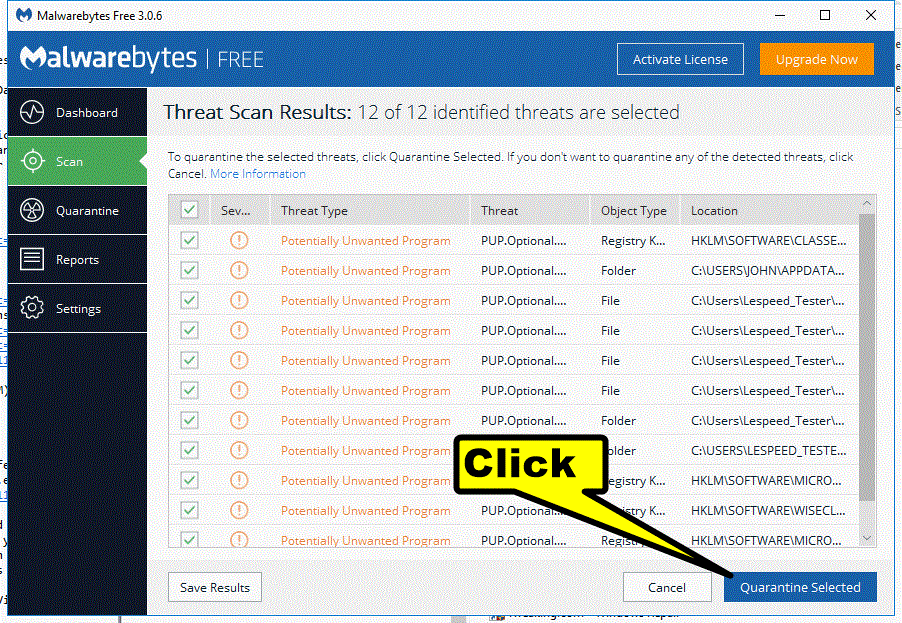
To reduce false positives, LogKernel further quantifies the threat of abnormal behaviour. In particular, we designed a new graph kernel clustering method based on the characteristics of BPGs, which can capture both structure information and rich label information of the BPGs. LogKernel first abstracts system audit logs into behaviour provenance graphs (BPGs) and then clusters graphs by embedding them into a continuous space using a graph kernel. In this paper, we propose LogKernel, a threat hunting method based on graph kernel clustering which can effectively separate attack behaviour from benign activities. However, most of the current threat hunting methods rely on Cyber Threat Intelligence (CTI), which can find known attacks but cannot find unknown attacks that have not been disclosed by CTI. It is a crucial component of active defense against advanced persistent threats (APTs). Cyber threat hunting is a proactive search process for hidden threats in an organization’s information system.
